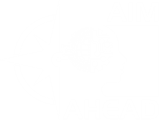
AIM-AHEAD AI/ML in Biomedical Research and Clinical Practice that Embodies Ethics and Equity (ABC-EE) Program
Cohort 1 Call for Proposals (Closed)
Key Dates:
- Solicitation Release Date: April 26, 2024
- Application Due Date: June 16, 2024 (11:59 EDT)
- Application Review Date: July 26, 2024
- Earliest Start Date: September 16, 2024
Informational Webinar:
Questions:
Please refer to the Frequently Asked Questions document before creating a help-desk ticket.
AI/ML in Biomedical Research and Clinical Practice FAQs
If your question is not answered in the above FAQ document, please create a help-desk ticket using the link below.
Issued by
Overview
The goal of the AIM-AHEAD AI/ML in Biomedical Research and Clinical Practice That Embodies Ethics and Equity (ABC-EE) program is to support multidisciplinary teams in the domains of biomedical research and clinical applications to develop and/or use AI/ML technologies that align with and/or extend the AIM-AHEAD ethics and equity principles [1]. This program will support teams at any stage of development, including those creating and/or validating frameworks for reasoning about the creation of AI/ML tools, on application of such tools in biomedical or behavioral health research, and on their translation into clinical care in a socially responsible manner. Topics of interest for this program include but are not limited to: the co-creation of data and/or AI/ML tools with target populations; the development of privacy enhancing technologies to support the collection, sharing, and/or use of such diverse data in AI/ML; and investigations into the trustworthiness and reliability of AI/ML, including emerging technologies such as generative AI and chatbots.
Applicants are expected to propose projects that address emerging issues to reduce health disparities while improving healthcare and outcomes in populations that experience health disparities in the United States.
[1] Hendricks-Sturrup R, et al. Developing ethics and equity principles, terms, and engagement tools to advance health equity and researcher diversity in AI and machine learning: modified delphi approach. JMIR AI. 2023; 2: e52888.
Background
The National Institutes of Health’s AIM-AHEAD program has established mutually beneficial, coordinated, and trusted partnerships to promote broad participation in the development of AI/ML models and to improve the capabilities of this emerging technology, beginning with electronic health records (EHR) and extending to other diverse data to address health disparities and inequities. Led by the AIM-AHEAD Coordinating Center, the consortium of institutions and organizations maintain as its core mission to serve underserved groups impacted by health disparities.
The rapid increase in the volume of data (e.g., in EHRs, public health activities, and biomedical and social science research) about factors that contribute to health and disease presents exciting opportunities for developing data science approaches (e.g., AI/ML methods) for improving healthcare. Many challenges hinder more widespread use of AI/ML technologies, such as the cost, capability for and acceptability of widespread application, and access to appropriate infrastructure, resources, and training. Additionally, without focuses on including representative data and promoting diversity in the AI/ML field, the field runs the risk of creating and perpetuating harmful biases in its practice, algorithms, and outcomes, thus fostering continued health disparities and inequities and undermining trust. Many underrepresented and underserved communities, which are often disproportionately affected by diseases and health conditions, have the potential to contribute expertise, perspectives, data, recruitment and engagement strategies, and cutting-edge science to inform the field on the most urgent research questions. Many communities and institutions, however, often lack financial, infrastructural, and data science training capacity to apply AI/ML approaches to research questions of interest to them. This program also seeks to build trust with individuals and groups affected by AI/ML.
AIM-AHEAD is committed to leveraging the potential of AI/ML to accelerate the pace of biomedical innovation, while prioritizing and addressing health disparities and inequities. Tackling the complex drivers of health disparities and inequities requires building a culture of innovative and transdisciplinary collaboration that transcends scientific and organizational silos and the status quo. Mutually beneficial and trusted partnerships can be established to encourage broad participation in AI/ML modeling and application and to improve capabilities for data curation and this emerging technology.
Please note that consistent with NIH practice and applicable law, funded programs may not use the race, ethnicity, or sex of prospective program participants or faculty as an eligibility or selection criteria. The race, ethnicity, or sex of candidates will not be considered by NIH in the application review process or when making funding decisions.
Purpose/Objectives
A major commitment of AIM-AHEAD is ensuring that AI/ML address health disparities and inequities and increase acceptance of these tools to improve healthcare access and health outcomes for populations that experience health disparities. Achieving these outcomes requires adherence to ethics and equity principles and building capacity among investigators and communities from diverse backgrounds. The primary objectives of this solicitation are to support: (a) multidisciplinary research projects that assess and enhance the role of ethics and equity in AI/ML for health and (b) projects that develop and implement strategies to overcome ethics and equity challenges.
Through this program, AIM-AHEAD envisions research projects that will help AI/ML research collaborators to:
- Better understand how decisions are made throughout the development of AI/ML, focusing on:
- How fully issues of ethics and equity are identified and addressed at all phases;
- The efficacy and timeliness of engagement with participants and communities in promoting ethics and equity and decreasing health disparities; and
- Identifying and overcoming institutional challenges in conducting AI/ML research;
- Better understand how AI/ML can be used to detect and mitigate bias in AI/ML models to avoid perpetuating, automating, and/or exacerbating bias and unfairness and reduce health disparities; and
- Improve healthcare and health outcomes.
Proposals to this program must include a plan for ensuring work is guided by a concern for human rights and social impacts, and attention to ethical, legal, and socio-economic implications of AI/ML, including but not limited to: (1) biases in datasets, algorithms, and applications; (2) issues related to identifiability and privacy; (3) impacts on disadvantaged or marginalized groups; (4) health disparities; and (5) unintended or adverse social, individual, and community consequences of development of implementation and research on AI/ML solutions.
Applicants are encouraged to propose projects that consider ethical issues and potential unintended adverse outcomes of bias and other issues in applying AI/ML approaches to improving health outcomes. It is expected that proposals will most likely be successful if they are engaged in and examining existing AI/ML research, whether collecting data prospectively or using retrospective data given the time frame. Applications that address freestanding normative and/or legal analyses that promise to provide new knowledge are within the scope of this program as well. Examples of the types of research projects that may be supported include but are not limited to:
- Examining the extent and impact of community engagement on data collected and/or research design for the creation or application of AI/ML
- Examining the extent and impact of inclusive research teams on research design and conduct
- Developing strategies for continual assessment of ethical and equity issues throughout the design, development, and use of AI/ML
- Documenting institutional challenges or barriers that arise during the course of research on AI/ML and, if applicable, steps that you took to address them
- Developing freestanding normative or legal analyses, for example,
- To what extent should community engagement be involved with existing datasets including the collection and interpretation of data?
- Evaluating evolving policy guidance regarding regulation of AI/ML or privacy protection on federal and/or state levels
- Developing human-centered and/or values-sensitive approaches to the creation and application of AI/ML
- Creating and/or evaluating methodologies that detect and/or mitigate biases in AI/ML
The NIH defines health disparities as differences in the incidence, prevalence, morbidity, mortality, and burden of diseases and other adverse health outcomes among specific population groups. Research projects should focus on one or more NIH-designated health disparity populations in the United States. These population groups include racial and ethnic minorities (African Americans, American Indians, Alaska Natives, Asian Americans, Hispanic Americans, Native Hawaiians, and other U.S. Pacific Islanders, as well as subpopulations of all of these racial/ethnic groups), socioeconomically disadvantaged individuals, sexual and gender minorities, underserved rural communities, and people with disabilities (see https://www.nimhd.nih.gov/about/strategic-plan/nih-strategic-plan-definitions-and-parameters.html).Research projects that include populations that identify across more than one subpopulation with and/or focus on intersectional effects on health disparities are encouraged.
Eligible Organizations
Consistent with the goals of the AIM-AHEAD Coordinating Center, the following types of Higher Education and other institutions/organizations are highly encouraged to apply for support:
Higher Education Institutions
- Public/State Controlled Institutions of Higher Education
- Private Institutions of Higher Education
The following types of Higher Education Institutions are always encouraged to apply for NIH support as Public or Private Institutions of Higher Education:
- Hispanic-serving Institutions
- Historically Black Colleges and Universities (HBCUs)
- Tribally Controlled Colleges and Universities (TCCUs)
- Alaska Native and Native Hawaiian Serving Institutions
- Asian American Native American Pacific Islander Serving Institutions (AANAPISIs)
Nonprofits Other Than Institutions of Higher Education
- Nonprofits with 501(c)(3) IRS Status
- Nonprofits without 501(c)(3) IRS Status
- Tribal health and/or human service organizations or Tribally derived institutions (Urban Indian Health Organizations, Tribal Epidemiology Centers)
- Small Businesses
- For-Profit Organizations (Other than Small Businesses)
To be eligible for this CFP, the applicant institution must be a domestic institution located in the United States and its territories which meet both of the following requirements:
- Has received an average of less than $50 million per year total costs of NIH support for the past three fiscal years.
- This requirement can be checked online on https://report.nih.gov/award/index.cfm.
- Follow this quick tutorial here to check your institutional NIH support.
- The administrative review (before scientific review) will check this requirement.
- Except for community organizations, nonprofits and non-academic institutions, has a documented historical mission to educate students from any of the populations that have been identified as underrepresented in biomedical, behavioral and social science research as defined by the National Science Foundation NSF, see http://www.nsf.gov/statistics/wmpd/) (i.e., African Americans or Blacks, Hispanic or Latino Americans, American Indians, Alaska Natives, Native Hawaiians, U.S. Pacific Islanders, and persons with disabilities) or has a documented historical track record of:
- Recruiting, training and/or educating, and graduating underrepresented students as defined by NSF (see above),
- Working with community stakeholders (e.g., Community-based organizations, Nonprofits (with or without 501(c)(3) IRS status, Faith-based organizations, Healthcare Providers, Health Systems, Small businesses, Large businesses, start-ups.) that have historically not participated in biomedical, behavioral, and social sciences research in the areas of AIM/ML
Community organizations, Nonprofits and Non-academic institutions are strongly encouraged to apply but should have a documented interest in working with health disparity populations. Before applying, these organizations must be registered with System for Award Management (SAM; see https://sam.gov/content/home) and must maintain active SAM registration throughout the award period.
Foreign Institutions
Non-domestic (non-U.S.) Entities (Foreign Institutions) are not eligible to apply.
Non-domestic (non-U.S.) components of U.S. Organizations are not eligible to apply.
Foreign components, as defined in the NIH Grants Policy Statement, are not allowed.
Required Registrations
Applicant organizations
Applicant organizations must complete and maintain the following registrations as described in the SF 424 (R&R) Application Guide to be eligible to apply for or receive an award. All registrations must be completed prior to the application being submitted. Registration can take 6 weeks or more, so applicants should begin the registration process as soon as possible. The NIH Policy on Late Submission of Grant Applications states that failure to complete registrations in advance of a due date is not a valid reason for a late submission.
- System for Award Management (SAM)Applicants must complete and maintain an active registration, which requires renewal at least annually. The renewal process may require as much time as the initial registration. SAM registration includes the assignment of a Commercial and Government Entity (CAGE) Code for domestic organizations which have not already been assigned a CAGE Code.
- NATO Commercial and Government Entity (NCAGE) Code Foreign organizations must obtain an NCAGE code (in lieu of a CAGE code) in order to register in SAM.
- Unique Entity Identifier (UEI) - A UEI is issued as part of the SAM.gov registration process. The same UEI must be used for all registrations, as well as on the grant application.
- eRA Commons- Once the unique organization identifier is established, organizations can register with eRA Commons in tandem with completing their Grants.gov registration; all registrations must be in place by time of submission. eRA Commons requires organizations to identify at least one Signing Official (SO) and at least one Program Director/Principal Investigator (PD/PI) account in order to submit an application.
- Applicants must have an active SAM registration in order to complete the Grants.gov registration.
Program Directors/Principal Investigators (PD(s)/PI(s))
All PD(s)/PI(s) must have an eRA Commons account. PD(s)/PI(s) should work with their organizational officials to either create a new account or to affiliate their existing account with the applicant organization in eRA Commons. If the PD/PI is also the organizational Signing Official, they must have two distinct eRA Commons accounts, one for each role. Obtaining an eRA Commons account can take up to 2 weeks.
Application Submission Guidelines, Components, and Review Process
Application Milestones
Milestones |
Deadlines |
Application Open |
04/26/2024 |
Application Submission Deadline |
06/16/2024 |
Reviews Complete |
08/30/2024 |
Notice of Award Dispatch |
09/03/2024 |
Program Start |
09/16/2024 |
Required Format:
- Arial font and no smaller than 11 point; margins at least 0.5 inches (sides, top and bottom); single-spaced lines. Submit the application as a single Word or pdf document to the application portal here.
Required Elements of the Proposal
- Title: The title should describe the project in the concise, informative language.
- Project Summary/Abstract (1-page limit): Provide a succinct description of the proposed work including the project’s long-term objectives, and a description of the research design and methods for the entire AI/ML project.
- Project Description: The project description should contain the following components adhering to the page limits.
- Specific Aims (1 page): Provide a clear, concise summary of the aims of the work proposed and its relationship to your long-term goals. State the hypothesis to be tested.
- Research Plan (5 pages)
- Background and Significance: Sketch the background and problem of the statement leading to this proposal. Summarize important results outlined by others in the same field, critically evaluating existing knowledge. Identify gaps that this project is intended to fill. State concisely the importance and relevance of the research to AI and health disparities research. Describe the health disparity population and ethical issues of interest. Also, it is incumbent upon the applicant to make a clear link between the project and the mission of the AIM-AHEAD. The significance section will be assessed in terms of the potential impact on the AIM-AHEAD mission; this will be factored into the overall priority score as noted in the peer review criteria.
- Preliminary Studies: Describe concisely previous work by the applicant related to the proposed research that will help to establish the experience and competence of the investigator to pursue the proposed project. Include pilot studies showing the work is feasible. (If none, so state.)
iii. Research Design and Methods: Description of proposed tests, methods or procedures should be explicit, sufficiently detailed, and well-defined to allow adequate evaluation of the approach to the problem. Describe any new methodology and how your research idea innovates or has an advantage over existing methods/applications and brings together diverse disciplines. Clearly describe the overall design of the study, with careful consideration to AI/ML and health disparities aspects of the approach or, and how your methods will control for bias and ethics, as well as how results will be analyzed. Include details of any collaborative arrangements that have been made. Applicants must explain how relevant social determinants of health and biological variables, such as sex, are factored into the research design, analysis and reporting. Furthermore, describe the infrastructure modality that would be used, data sources needed and used, compute infrastructure needed and used (see data and infrastructure section). Describe plan for ensuring work is guided by a concern for human and social impact and attention to ethical, legal, and social implications of AI/ML including but not limited to (1) ethics and equity, (2) biases in datasets, algorithms, and applications; (2) privacy, security, and trust; (3) impacts on disadvantaged or marginalized groups; (4) health disparities; and (5) unintended, adverse social, individual, and community consequences of research and development.
- Data: Applicants are required to describe (1) reference(s) to the data under consideration and reasons for this choice; (2) the potential impact of scientific advances that could be made from AI/ML applications developed with the data; (3) proposed methods/data modalities to be used; (4) how the data will be made available to AI/ML applications and researchers, for example, through NIH repositories, NIH knowledge bases, or other data sharing resources including those appropriate for controlled access data; and (5) how the ethical implications of the data will be identified and addressed.
- References Cited (maximum 3 pages):List only references cited in the Project Description or supplementary documents of the proposal.
- Detailed Budget and Budget Justification: Funding Amounts
In order to ensure that the AIM-AHEAD –Coordinating Center is able to fund substantial projects, the budget for the projects will be in the range of $150,000 to $250,000 in total costs. The total cost includes indirect cost allowances. It is possible that the budget for the projects could be higher (with appropriate justification) or lower than this estimate based on the resources needed to complete the project.
Funding Period
Applicants may request up to 1 year of funding support commensurate with project scope.
Budget: Year One
Personnel |
Base Salary |
Months Effort |
Requested Salary |
Fringe |
Total Funds Requested |
Principal Investigator: |
$ |
|
$ |
$ |
$ |
MPI: |
$ |
|
$ |
$ |
$ |
Co-Investigator: |
$ |
|
$ |
$ |
$ |
Co-Investigator: |
$ |
|
$ |
$ |
$ |
Research Coordinator: |
$ |
|
$ |
$ |
$ |
Research Assistant |
$ |
|
$ |
$ |
$ |
Subtotal: Personnel |
$ |
|
$ |
$ |
$ |
|
|||||
Maintenance & Operation |
Funds Requested |
||||
Subject Payments |
$ |
||||
Vertebrate Animals |
$ |
||||
Consumable Supplies |
$ |
||||
Equipment |
$ |
||||
Travel |
$ |
||||
Subtotal: Maintenance & Operation |
$ |
||||
|
|||||
Total Direct Costs |
$ |
||||
Indirect Costs/F&A (xx% of Direct Costs) |
$ |
||||
Total Costs |
$ |
Allowable costs:
- Academic Institutions: The award may be used for salary and fringe benefits of the Co-Principal Investigators, collaborating investigator(s), and other participants with faculty appointments, commensurate with percent effort, and for project-related expenses, such as salaries of technical personnel essential to the conduct of the project, supplies, equipment, computers/electronics, travel (including international travel), volunteer subject costs, data management, publication costs, etc. Tuition support for graduate students may also be requested.
- Other non-Academic Institutions/Organizations: The award may be used for salary and fringe benefits of the Principal Investigators, collaborating investigator(s), and other participants consistent with salary structure, and for project-related expenses, such as salaries of technical personnel essential to the conduct of the project, supplies, equipment, computers/electronics, travel (including international travel), volunteer subject costs, data management, publication costs, etc.
The budget justification should entail a narrative explanation of each of the components of the costs for performing proposed work. The budget explanations should focus on how each budget item is required to achieve the project’s objectives and how the estimated costs in the budget were calculated.
6. Facilities, Equipment and Other Resources: Facilities, Equipment and Other Resources should describe the resources needed and those that are available to for the proposed research project. Collaborators should also provide this information. Applicants should explain how well the scientific environment in which they will conduct their research contributes to the probability of success.
7. Senior Personnel Documents:
- Biographical Sketches: Biographical sketches are required for the PI, any co-PIs, and each of the participating Senior Personnel listed in the Project Description (including postdocs, staff, and /or students). All biographical sketches submitted in response to this solicitation are expected to follow the NIH format. Biosketch Format Pages, Instructions and Samples | grants.nih.gov
- Current and Pending Support: Include any funding in the last three years from NIH or AIM-AHEAD or Federal sources.
- Collaborators and Other Affiliations Information.
8. Human Subjects Research.
- Does any of the proposed research involve human subjects and/or data (Including EHR and/or repository data)? Describe how such subjects and/or data are or are not regulated by the Common Rule
- Does your research require a Data Use Agreement or other agreement(s) for use of data?
- If yes, what is the plan to execute these agreements among all partners/collaborators?
Note: Definition of Human subject: A living individual about whom an investigator (whether professional or student) conducting research: (1) obtains information or biospecimens through intervention or interaction with the individual, and uses, studies, or analyzes the information or biospecimens; or (2) obtains, uses, studies, analyzes, or generates identifiable private information or identifiable biospecimens. [45 CFR 46.102(e)].
Required Training/Certifications
Awardees will be expected to complete Human Subjects Research training (e.g., CITI or the free training offered by OHRP found at https://www.hhs.gov/ohrp/education-and-outreach/human-research-protection-training/human-research-protection-foundational-training/index.html) and any other training required by datasets they intend to use in their projects.
9. Other Supplementary Documents:
- Institutional Need and Support Statement (up to 3 pages). This statement must be signed by the leadership of the institution (e.g., University president, provost or college/school dean as applicable to show support for the partnership and commitment to additional resources necessary to ensure that these partnerships will have the maximum sustainability. This letter should include
- Assessment of the institution’s current AI research, education as well as data and infrastructure capacity;
- A statement of commitment of institutional support for the proposed activities. List the specific resources, space, protected time, etc. These statements should also identify the specific number of positions that are wholly dedicated to AI data and infrastructure under the partnership. In addition, if American Indians are involved, a Letter of Commitment from the Tribal Nation Leader is required.
- Letter from Sponsor Office: This letter should indicate that your institution has received an average of less than $50 million per year total costs of NIH support for the past three fiscal years.
- Results from Prior NIH/AIM-AHEAD Support.If applicable, you must submit the Results from Prior NIH/AIM-AHEAD Support as a supplementary document, rather than as part of your Project Description. This allows you to maximize the use of the page allowance to describe the proposed activities.
- Cloud Computing Resources (if applicable).
- Project Personnel and Partner Organizations (required).
Review Process
A Review Committee composed of AIM-AHEAD Consortium members will oversee the following steps and criteria to evaluate proposals and recommend award recipients to NIH, which makes the final decision on awards.
Proposals will be reviewed with respect to their consideration of:
- Human-centered design of AI/ML
- Multidisciplinary approaches to the creation and application of AI/ML
- Community engagement
- Access to data, algorithms, and software
- Health disparities
- Algorithmic fairness
- Privacy, security, and trust
- Attention to ethics and equity
- Legal issues where appropriate
Assessment of scientific merit will be guided by the specific questions related to the proposed project:
- How well does the project collect empirical evidence about the application of ethics and equity principles in the design, development, and/or use of AI/ML and their impact on addressing health disparities?
- How well does the project collect information about challenges, inclusive of ethics and equity issues, in the design, development, and/or use of AI/ML and their impact on addressing health disparities in their institution?
- Does the team describe plans to identify, develop, and evaluate strategies to overcome any challenges observed?
- To what extent does the proposal appropriately consider ethical, legal, and privacy considerations?
- To what extent the proposal describes how the selected dataset(s) will contribute to addressing the research topic and approaches and/or creating tools that will benefit the functionality of the AIM-AHEAD ecosystem?
- How well does the proposal clearly articulate the goals of the project and expected outcomes in terms of research products or other artifacts?
- To what extent is the proposed approach reasonably likely to achieve the goals of the project? What is the likelihood of a successful outcome? Are the measures of success clearly defined?
- Does the team have the necessary background and capabilities to accomplish the proposed work in the expected funding period?
A Review Committee composed of AIM-AHEAD Consortium members will evaluate proposals and recommend award recipients to NIH, which makes the final decision on awards.
Compliance review: Program staff will do an initial check of applications for compliance with requirements of this Call for Proposals. Non-compliant applications may be rejected or require remediation prior to Scientific Review and Programmatic Evaluation.
Scientific evaluation: A panel of reviewers will apply standard NIH scoring ranges to each application on each of the nine scientific merit criteria listed above.
Programmatic evaluation:
It is expected that approximately 10 awards will be assigned to the highest-scoring applications based on Scientific Merit and that the rest of the awards could be, but not necessarily will be, recommended for NIH consideration based on both Scientific Merit scores and Programmatic Evaluation considerations. The consortium will take steps to avoid any conflicts of interest with reviewers or voting MPIs. This is particularly important because this CFP allows for participation of AIM-AHEAD Hub or Core partners in the projects.
Applicants will receive their scores and notes from the Scientific Merit reviewers.
Awardee Expectations
Applicants are encouraged to propose projects that consider ethical issues and potential unintended adverse outcomes of bias in applying AI/ML approaches to improving health outcomes. It is expected that proposals will most likely be successful if they are engaged in and examining existing AI/ML research, whether collecting data prospectively or using retrospective data, given the time frame. Applications that address freestanding normative and/or legal analyses that promise to provide new knowledge are within the scope of this program as well. Therefore, awardees are expected to fulfill the following expectations:
Participation and Reports
- Awardees will participate in regular awardee virtual meetings.
- Awards will submit bimonthly reports and budget reports.
- Awardees will participate in meetings with the AIM-AHEAD Coordinating Center to inform overall AIM-AHEAD data sharing / data access strategies.
- Awardees will act as AIM-AHEAD community ambassadors to help onboard colleagues.
- Awardees must be willing to attend and present the results of their work at future AIM-AHEAD events as well as volunteer to review applications for future AIM-AHEAD programs.
- Awardees agree to have AIM-AHEAD promote the project online through websites, social media, and other communication channels.
- All awardees will be expected to be involved in AIM-AHEAD activities during the year, including attending the in-person annual meetings.
- Awardees must provide a summary of research status about milestones listed in the proposal, challenges faced and plans to overcome those challenges, usage of funds, and next steps.
- By one month after the project end date, awardees must provide a final report of research findings, use of funds, and a list of publications, grant applications, articles, and conference talks emerging from the research.
Compliance and Governance
- For all research projects involving human subjects research (including secondary data analysis), awardees are expected to submit their study for review to an IRB and obtain a determination or letter/response (See section Application Components section, subsection 10). **Even if the projects do not involve human subjects, a “letter of determination” is required. When a local IRB is not available, alternative options should be used.
- Awardees are required to obtain any required Data Use/Sharing or Regulatory/Contractual Agreements for data needed for their research.
- Any current or future data sharing must follow relevant governance documents and agreements.
- Awardees must comply with all applicable Federal statutes (such as those included in appropriations acts), regulations, and policies in addition to their institutional and state policies to receive research funding.
Note: No funds can be drawn down from NIH payment system, and no obligations may be made by any site of AIM-AHEAD’s coordinating center for research for any period not covered by both an OHRP-approved Federal Wide Assurance and approval from the IRB, as required, consistent with 45 CFR Part 46 and any NIH required policies for research involving human subjects.
Ethical Use of Data
The Applied Ethical AI (AEAI) Subcore was established to provide support in surfacing, reasoning about, and resolving ethical issues in the AIM-AHEAD program. It is expected that all projects will work with the AEAI on the development of an initial ethics review within the first 4-6 weeks of support and will commit to participating in two AEAI-supported ethics forums during the course of their sponsorship. These forums are designed to highlight and address ethical challenges in the development and implementation of AI and machine learning and range from open office hours to moderated discussions on hot topics in ethics and AI/ML. Topics that may be addressed during these forums include but are not limited to:
- Investigation into the ethical grounding of the project and the plans for embedding ethical reasoning into the project.
- Consultation on how to test for algorithmic fairness and data bias with respect to potentially sensitive variables (e.g., socio-demographics)
- Detection of potential data privacy concerns and alternatives for their resolution
Sharing of research products
AIM-AHEAD Service Workbench Data Repository
The AIM-AHEAD Service Workbench platform https://aim-ahead.net/swb is a FISMA (Federal Information Security Modernization Act) secure environment working towards Authority to Operate (ATO) status that can serve as a repository for the data sharing needs of AIM-AHEAD projects and members. The AIM-AHEAD Service Workbench Centralized FISMA secure Infrastructure can provide secure hosting of data, management of access controls with appropriate authentication and authorization and continuous monitoring of the centralized environment that ensures system cybersecurity. More information in this document from NIH on how AIM-AHEAD repository was established: Establishing a Controlled Access Repository for AIM-AHEAD
AIM-AHEAD will assist awarded investigators to identify an appropriate data repository, prepare data for transfer, and meet requirements of the data repository.
Questions
Please direct questions regarding the ABC-EE program to applicant support at Carolyn.diehl@vumc.org or Laurie.l.novak@vumc.org.
Answers to frequently asked questions are posted here.
Awardee Resources
Glossary from Developing Ethics and Equity Principles, Terms, and Engagement Tools to Advance Health Equity and Researcher Diversity in AI and Machine Learning: Modified Delphi Approach, R. Hendricks-Sturrup, M. Simmons, S. Anders, K. Aneni, E. W. Clayton, J. Coco, et al., JMIR AI 2023 Vol. 2 Issue 1 Pages e52888
No. |
Glossary Term |
AIM-AHEAD Definition |
1 |
Ethnicity |
Distinct patterns of language, lifestyle, illness, and health beliefs encountered among an individual or representative population, regardless of race, and that may subject the individual or population to bias or discrimination. |
2 |
Race |
A social construct or assumption based on patterns in an individual’s or representative population’s language, lifestyle, and/or health beliefs, and immutable characteristics, such as skin tone/color or hair texture, regardless of immigration status, socioeconomic status, genetic ancestry, or geographic origin, which may subject the individual’s or population to bias, structural racism, and/or discrimination that would warrant corrective anti-racism action(s). |
3 |
Bias |
Systematic error in information originating, gathering, or assessment activities, leading to selecting or encouraging one outcome or answer over others, which can result in human decisions and values that echo societal or historical inequities, and/or produce inconclusive or limited assumptions about the broader population. |
4 |
Equity |
Equity is fairness and justice in policy, practice, and opportunity designed to address the distinct challenges of non-dominant social groups, with an eye to progressive outcomes. Health equity is the state in which everyone has the opportunity to attain full health potential and no individual is disadvantaged from achieving this potential because of social position or any other socially defined circumstance. |
5 |
Algorithmic Bias |
Systematic and repeated errors in the collection and consideration of a variety of factors, including but not limited to the design of the algorithm; unintended or unanticipated use or decisions relating to the way data are collected, represented, or used; lack of sensitivity to identity factors that contribute to bias in the evaluation of the algorithm, or misappropriation of the algorithm through miscommunicating or misunderstanding its limitations. |
6 |
Diversity |
The wide variety of shared and different personal and group characteristics among human beings. There are many kinds of diversity, including gender, sexual orientation, class, age, country of origin, education, religion, geography, physical or cognitive abilities, or other characteristics. Valuing diversity means recognizing differences between people, acknowledging that these differences are a valued asset, and striving for diverse representation as a critical step towards equity. |
7 |
Inclusive |
Avoiding bias by providing equitable and open access to opportunities and resources for engagement. This can be accomplished, for example, by enforcing fairness in the data collection methods, enforcing fairness in the assignment of labels, developing explainable, transparent, and interpretable models, having diverse teams monitoring models and looking for biases and eliminating them. |
8 |
Fairness |
Intent to promote nondiscrimination and population representation when assessing a group’s eligibility for a benefit or penalty. This is particularly important given the statistical likelihood that AI/ML systems could produce discriminatory outputs once algorithms are implemented across one or more datasets. |
9
|
Representative
|
An individual or body chosen or appointed to act or speak for an individual, population, or subpopulation sharing a set of features or characteristics, including but not limited to gender, race, and/or sexual orientation. |
10 |
Representative Sample |
A subset of a population that reflects the characteristics of the entire population from which it has been selected. |
11 |
Gender Identity |
An individual’s sense of oneself as male, female, or something else. When an individual’s gender identity and biological sex are not congruent, the individual may identify along the transgender spectrum. An individual may choose to change their gender one or more times. Varying cultural indicators of gender, such as clothing choice, speech patterns, and personality traits relate to gender but are not acceptable means to determine another’s gender identity. The change in an individual’s gender can be used to abuse, discriminate against, and misrepresent individuals and groups. |
12 |
Sexual Orientation |
An individual’s capacity for attraction to and sexual activity with the same or different sex. An individual’s sexual orientation is indicated by one or more of the following: how an individual identifies their own sexual orientation, an individual’s capacity for experiencing sexual and/or affectional attraction to people of the same and/or different gender, and/or an individual’s sexual behavior with people of the same and/or different gender. Sexual orientation incorporates three core ideas: consensual human relationships—sexual, romantic, or both—the biological sex of an individual’s actual or potential relationship partners, and enduring patterns of experience and behavior. Sexual minorities, or people whose sexual orientation does not conform to heteronormative cultural expectations, are vulnerable to violence and discrimination. |
AIM-AHEAD consists of its Leadership Core made up of Regional Hubs and the following Cores:
- Data Science Training Core (DSTC)
- Data and Research Core (DRC),
- Infrastructure Core (IC) and its Applied Ethical AI (AEAI) Subcore
Data sources and accessibility
Proposed projects may either identify their own datasets and/or be approved for use of existing AIM-AHEAD resources, including the OCHIN Community Health Equity Database on AIM-AHEAD Service Workbench or MedStar Health EHR through the AIM-AHEAD Data Bridge (AADB).
Requirements Prior to Obtaining Access to AIM-AHEAD Curated Data (OCHIN/AADB)
- Mandatory Human Subjects Research Training such as CITI “Human Research (Protection of Human Subjects)” and “Responsible Conduct of Research.”
- Data consult with the data source (i.e., OCHIN or MedStar) to finalize data request within 30 days of award.
- Submission for IRB approval/determination within 60 days of award.
- Signed data use agreement and IRB approval/determination within 90 days of award.
Dataset options (more information available on below datasets/cohorts):
Data set |
Brief Description |
Data Allowed |
Size |
Analysis platform tools |
A customized subset from OCHIN Community Health Equity Database |
EHR data from Underserved communities |
HIPAA Limited dataset, individual-patient level data with dates and geographic indicators if needed for research |
A customized subset will be created for the research question of awarded fellow from over 6 million records |
|
EHR data from hospital system network with 31% African American patient representation |
Multiple curated dataset options (further detail on website) pre-curated or custom curated de-identified EHR, Limited Dataset, Full PHI EHR dataset, Imaging, Select clinical notes, select genomics data, synthetic data |
Pre-curated datasets and custom-curated datasets of varying sizes
Curated from the EHR with over 5 million patient records |
||
Selected large-scale cohorts related to heart, lung, blood and sleep disorders. Includes both prospective clinical studies and associated genomic TOPMED data. |
De-identified dataset. Including individual level genomic (TOPMED full genomes) and clinical datasets. |
List of studies: 60+ studies are available to choose from |
NHLBI BioData Catalyst PIC-SURE and Seven Bridges Platforms |
|
A variety of datasets available including clinical and genomic data |
Public data, and controlled access data (depends on dataset) |
|||
The All of Us Research Program is building one of the largest biomedical data resources of its kind. |
The All of Us Research Hub stores health data from a diverse group of participants from across the United States.
|
Additional descriptions Participants
360,000+ Electronic Health Records
444,000+ Biosamples Received |
Dataset Infrastructure
Infrastructure of 1) OCHIN and 2) AWS Open Datasets will use AIM-AHEAD Service Workbench (SWB). SWB is a tool for any cloud computing data analysis that will be conducted throughout this fellowship. More information is available here.
Infrastructure for BioData Catalyst will use the NHLBI BioData Catalyst PIC-SURE and Seven Bridges Platforms. An NIH eRA Commons ID in order to access these resources.
Infrastructure for AIM-AHEAD Data Bridge will connect to a Windows Virtual Machine (VM).
Infrastructure for All of Us Research Program will use the All of Us Workbench which is a cloud-based platform where registered researchers can access Registered and Controlled Tier data.
OCHIN Community Health Equity Database
Applicants may want to explore the OCHIN Community Health Equity Database for feasibility assessment as they write their proposals. OCHIN provides the means to do so through the use of Cohort Discovery, a web-based software tool for obtaining counts of patients matching user-specified inclusion/exclusion criteria.
To gain access to Cohort Discovery, AIM-AHEAD program applicants must have completed and be up to date with standard training in Human Subjects Research and Responsible Conduct of Research such as those offered by the CITI Program. To request access to OCHIN’s Cohort Discovery, AIM-AHEAD program applicants can complete the OCHIN i2b2 End User Agreement.
AIM-AHEAD DataBridge
Applicants whose research question can be addressed with AIM-AHEAD Data Bridge (AADB) data may choose to schedule a pre-award data consultation with AADB to discuss their research question with the AADB team and methodologist. The AADB can perform a preliminary cohort discovery to provide estimated sample sizes for custom dataset requests.
Available EHR data including (but not limited to): demographics, problems list, surgical history, observations, medical history, procedures, prescriptions, billing, provider, clinical notes, clinical unit/department, allergies, images, social history, diagnoses, admissions, encounters, social history, social determinants of health, device implants
NHLBI BioData Catalyst studies
Over the last several decades, NHLBI has invested in creating a significant resource for research and development by supporting the creation of many observational, epidemiological, and longitudinal datasets related to heart, lung, blood and sleep phenotypes, with the aim of uncovering insights that may be leveraged toward novel therapeutic, interventional, or preventive strategies resulting in improved patient outcomes. New technologies and favorable cost trajectories have enabled detailed characterization of these study participants, including whole genome sequencing (and other omics) and imaging on hundreds of thousands of participants. Together, this data coupled with animal and cellular models, increase opportunities for data-driven translational science. We have fully entered the “Big Data” arena, in which we encounter both unprecedented opportunities as well as challenges. Current paradigms for analyzing and combining these datasets are limited by both practical and conceptual constraints. The NHLBI BioData Catalyst, is a novel ecosystem of platforms and tools to enable and accelerate the mining of these rich datasets from diverse populations. Full Description (including patient, variables, and sample counts ) is available here.
Acronym |
Name |
Study Focus |
Study Design |
FHS |
Framingham Cohort |
Cardiovascular Disease |
Prospective Longitudinal Cohort |
JHS |
Jackson Heart Study (JHS) Cohort |
Cardiovascular Disease |
Prospective Longitudinal Cohort |
CARDIA |
Coronary Artery Risk Development in Young Adults (CARDIA) |
Cardiovascular Disease |
Prospective Longitudinal Cohort |
ARIC |
NHLBI TOPMed - NHGRI CCDG: Atherosclerosis Risk in Communities (ARIC) |
Cardiovascular Disease |
Case-Control |
WHI |
Women's Health Initiative Clinical Trial and Observational Study |
Women's Health |
Prospective Longitudinal Cohort |
ACTIV4a |
A Multicenter, Adaptive, Randomized Controlled Platform Trial of the Safety and Efficacy of Antithrombotic Strategies in Hospitalized Adults with COVID-19 (ACTIV4A) |
COVID-19 |
Interventional |
ACTIV4b |
COVID-19 Positive Outpatient Thrombosis Prevention in Adults Aged 40-80 |
COVID-19 |
Interventional |
AMISH |
NHLBI TOPMed: Genetics of Cardiometabolic Health in the Amish |
Cardiovascular Disease |
Family/Twin/Trios |
BABYHUG |
Hydroxyurea to Prevent Organ Damage in Children with Sickle Cell Anemia (BABY HUG) Phase III Clinical Trial and Follow-Up Observational Studies I and II |
Sickle Cell Anemia |
Clinical Trial |
BAGS |
NHLBI TOPMed: The Genetics and Epidemiology of Asthma in Barbados |
Asthma |
Family/Twin/Trios |
C3PO |
Clinical-trial of COVID-19 Convalescent Plasma in Outpatients |
COVID-19 |
Clinical Trial |
CATHGEN |
CATHeterization GENetics (CATHGEN) |
Coronary Disease |
Cross-Sectional |
CCAF |
The Cleveland Clinic Foundation's Lone Atrial Fibrillation GWAS Study |
Atrial Fibrillation |
Case Set |
CFS |
NHLBI Cleveland Family Study (CFS) Candidate Gene Association Resource (CARe) |
Sleep Apnea Syndromes |
Prospective Longitudinal Cohort |
COPDGENE |
Genetic Epidemiology of COPD (COPDGene) |
Pulmonary Disease, Chronic Obstructive |
Case-Control |
CRA |
NHLBI TOPMed: The Genetic Epidemiology of Asthma in Costa Rica |
Asthma |
Family/Twin/Trios |
CSSCD |
Cooperative Study of Sickle Cell Disease (CSSCD) |
Sickle Cell Disease |
Clinical Trial |
DHS |
NHLBI TOPMed: Diabetes Heart Study (DHS) African American Coronary Artery Calcification (AA CAC) |
Cardiovascular Disease |
Cross-Sectional |
ECLIPSE |
Evaluation of COPD Longitudinally to Identify Predictive Surrogate Endpoints (ECLIPSE) |
Pulmonary Disease, Chronic Obstructive |
Case-Control |
EOCOPD |
NHLBI TOPMed: Boston Early-Onset COPD Study |
Chronic Obstructive Pulmonary Disease |
Family/Twin/Trios |
GALAII |
Genes-Environments and Admixture in Latino Asthmatics (GALA II) Study |
Lung Diseases |
Case-Control |
GENESTAR |
GeneSTAR (Genetic Study of Atherosclerosis Risk) NextGen Consortium: Functional Genomics of Platelet Aggregation Using iPS and Derived Megakaryocytes |
Platelet Aggregation |
Prospective Longitudinal Cohort |
GENOA |
Genetic Epidemiology Network of Arteriopathy (GENOA) |
Hypertension |
Prospective Longitudinal Cohort |
GENSALT |
Genetic Epidemiology Network of Salt Sensitivity (GenSalt) |
Arterial Pressure, Mean |
Interventional |
GOLDN |
NHLBI TOPMed: GOLDN Epigenetic Determinants of Lipid Response to Dietary Fat and Fenofibrate |
Lipids |
Prospective Longitudinal Cohort |
HCHSSOL |
Hispanic Community Health Study /Study of Latinos (HCHS/SOL) |
Cardiovascular Disease |
Prospective Longitudinal Cohort |
HCT_for_SCD |
Hematopoietic Cell Transplant for Sickle Cell Disease (HCT for SCD) |
Sickle Cell Disease |
Prospective Longitudinal Cohort |
HVH |
Heart and Vascular Health Study (HVH) |
Cardiovascular Disease |
Case-Control |
HYPERGEN |
NHLBI TOPMed: HyperGEN - Genetics of Left Ventricular (LV) Hypertrophy |
Hypertrophy, Left Ventricular |
Family/Twin/Trios |
MAYOVTE |
NHLBI TOPMed: Whole Genome Sequencing of Venous Thromboembolism (WGS of VTE) |
Venous Thromboembolism |
Case Set |
MESA |
Multi-Ethnic Study of Atherosclerosis (MESA) SHARe |
Cardiovascular Disease |
Prospective Longitudinal Cohort |
MGHAF |
Massachusetts General Hospital (MGH) Atrial Fibrillation Study |
Atrial Fibrillation |
Case Set |
MSH |
Multicenter Study of Hydroxyurea (MSH) |
Sickle Cell Disease |
Clinical Trial |
NSRR-CFS |
National Sleep Research Resource (NSRR): Cleveland Family Study (CFS) |
Sleep Apnea Syndromes |
Prospective Longitudinal Cohort |
ORCHID |
COVID19-ORCHID |
COVID-19 |
Clinical Trial |
PARTNERS |
NHLBI TOPMed: Partners HealthCare Biobank |
Atrial Fibrillation |
Case Set |
PCGC |
The Pediatric Cardiac Genetics Consortium (PCGC) Study |
Heart Defects, Congenital |
Prospective Longitudinal Cohort |
RED_CORAL |
PETAL Repository of Electronic Data COVID-19 Observational Study (RED CORAL) |
COVID-19 |
Control Set |
SAFHS |
NHLBI TOPMed: San Antonio Family Heart Study (SAFHS) |
Cardiovascular Disease |
Family/Twin/Trios |
SAGE |
NHLBI TOPMed: Study of African Americans, Asthma, Genes and Environment (SAGE) Study |
Lung Diseases |
Case Set |
SARCOIDOSIS |
NHLBI TOPMed: African American Sarcoidosis Genetics Resource |
Sarcoidosis |
Family/Twin/Trios |
SARP |
NHLBI GO-ESP: Lung Cohorts Exome Sequencing Project (Asthma): Genetic variants affecting susceptibility and severity |
Asthma |
Case Set |
SAS |
Genome-Wide Association Study of Adiposity in Samoans |
Obesity |
Cross-Sectional |
SHARP |
SNP Health Association Asthma Resource Project |
Lung Diseases |
|
STOP-II |
Optimizing Primary Stroke Prevention in Children with Sickle Cell Anemia (STOP II) |
Sickle Cell Disease |
Clinical Trial |
THRV |
NHLBI TOPMed: Rare Variants for Hypertension in Taiwan Chinese (THRV) |
Blood Pressure |
Prospective Longitudinal Cohort |
VAFAR |
NHLBI TOPMed - NHGRI CCDG: The Vanderbilt AF Ablation Registry |
Atrial Fibrillation |
Case Set |
VUAF |
NHLBI TOPMed: The Vanderbilt Atrial Fibrillation Registry (VU_AF) |
Atrial Fibrillation |
Case Set |
Walk-PHaSST |
Treatment of Pulmonary Hypertension and Sickle Cell Disease with Sildenafil Therapy (Walk-PHaSST) |
Sickle Cell Anemia |
Clinical Trial |
WGHS |
NHLBI TOPMed: Novel Risk Factors for the Development of Atrial Fibrillation in Women |
Atrial Fibrillation |
Case Set |
AWS Open Datasets
More information (description and links) is available here
Acronym |
Name of AWS open dataset |
EMBED |
|
1000-genomes |
|
tcga |
|
broad-gnomad |
|
broad-pan-ukb |
|
kids-first |
Gabriella Miller Kids First Pediatric Research Program (Kids First) |
target |
Therapeutically Applicable Research to Generate Effective Treatments (TARGET) |
hcmi-cmdc |
Human Cancer Models Initiative (HCMI) Cancer Model Development Center |
cgci |
Cancer Genome Characterization Initiatives - Burkitt Lymphoma, HIV+ Cervical Cancer |
organoid-pancreatic |
|
nciccr-dlbcl |
|
mmrf-commpass |
|
hcp-openaccess |
|
cptac-2 |
|
cptac-3 |
NIH All of Us
The All of Us Research Program’s centralized, secure, cloud-based platform allows researchers across a wide range of settings and institutions and at all stages of their careers (e.g., students, early-stage investigators) to execute rapid, hypothesis-driven research with just a computer and an Internet connection.
All of Us facilitates equity in access in a deliberately inclusive way, creating a demographically diverse researcher cohort.
Currently, academic, not-for-profit and healthcare organizations are eligible to apply for an All of Us Data Use and Registration Agreement (DURA). This is the first step to accessing the All of Us Researcher Workbench.
Within the Researcher Workbench's Controlled Tier, data from nearly 250,000 whole genome sequences and more than 312,900 genotyping arrays are integrated alongside data from surveys, physical measurements, EHRs, and wearables. The data and tools are available only to registered researchers who have taken additional steps and training to access these data.
Submission using AIM-AHEAD Connect and InfoReady platform
- Step 1: Click here to login or to register as a “mentor" on AIM-AHEAD Connect (our Community Building Platform)
- Step 2: After you login to AIM-AHEAD Connect, click here to submit an application for review using the InfoReady platform*.
*To submit your application in InfoReady, please use Chrome, Firefox, or Edge. If you're using Safari, make sure to clear your cache before logging in.
Please note both steps must be completed for consideration.
All applications must be received by June 16, 2024 — 11:59 PM Eastern Time.