AIM-AHEAD Hosts Research Fellowship Cohort 3 Kickoff Call
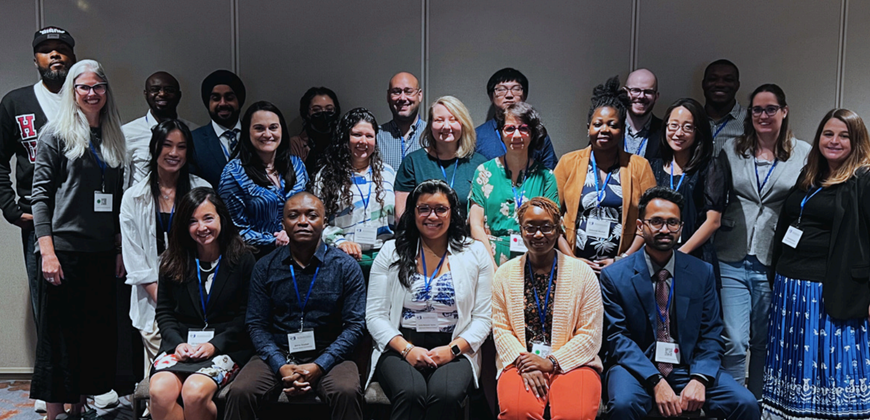
On Friday, October 4, 2024, the AIM-AHEAD Research Fellowship Program held a kickoff call for its Cohort 3 fellows. The Research Fellowship Program Leadership Team, consisting of Dr. Paul Avillach, Program Director; Dr. Wyatt Bensken, Co-Director; Dr. Nawar Shara, Co-Director, and Dr. Chunmei Lui, Co-Director, hosted the event.
The 25 fellows were recognized, and leadership gave an overview of the AIM-AHEAD Consortium, program expectations, and the data resources available to fellows in their research. The Research Fellowship Program is a 12-month program that engages early-career researchers to actively participate in biomedical research using artificial intelligence/machine learning (AI/ML) methodologies.
All fellows receive access to AIM-AHEAD data provided by OCHIN's Community Health Equity Database, AADB/MedStar EHR, BioData Catalyst, AWS, All of Us, and ScHARe, as well as infrastructure support. Each fellow will be matched with an AIM-AHEAD Mentor for the duration of the program, which ends on September 30, 2025.
Each fellow will complete the following goals throughout the year:
- Onboard to the AIM-AHEAD Research Fellowship Program
- Complete the necessary training to be successful in the fellowship program
- Actively participate in fellowship program meetings and deliver reports
- Collaborate with mentor(s) and program stakeholders to complete research goals
- Successfully conclude research fellowship and deliver a poster presentation with final findings
Research Fellowship Program Cohort 3 Fellows
(Name, Institution, Project Title)
- Pegah Ahadian, Kent State University, Utilizing EHR Data to Enhance Fairness in Mental Healthcare: A Comprehensive Analysis of Disparity Ratios, Timeliness of Care, and Preventive Care Rates in Depression Patient Populations
- Sadia Akter, Marshall University, Uncovering Asthma Disease Mechanisms in Latino and African American Populations by applying AI in Human multi-omics data
- Alaina Beauchamp, University of Texas Southwestern Medical Center, Using Spatial Machine Learning Algorithms to Predict Behavioral Health Management Among Multimorbidity Patients at Community Health Centers
- Yu-Lun Chen, Kessler Foundation, Clinical and Social Predictors of Mental Health Disparities in Adults on the Autism Spectrum
- Daniil Filienko, University of Washington Tacoma, Equitable AI for subtyping Acute Myeloid Leukemia
- Nathaniel Hendrix, American Board of Family Medicine, Causal Machine Learning for Identifying Equity-Improving Interventions in Type 2 Diabetes
- Alexandra Kakadiaris, Rice University, Healthy maternal hearts and healthy babies: Predicting peripartum cardiomyopathy in African American Women
- Kiyoung Kim, Texas A&M University, Improving Fairness in AI and Machine Learning-Based Risk Prediction of Physical Inactivity in Individuals with Hypertension
- Vijay Kunwar, Albany State University, An Analytical Study on the Prevalence of Diabetes among the African American Community in the United States
- Monique McCallister, Tennessee State University, Identification and Characterization of Factors/Variables Leading to Adverse Health Outcomes and Health Disparities in Residents Living in Low Socioeconomic Communities
- Natalia Maldonado Vazquez, University of Puerto Rico - Centro Comprensivo de Cancer, Epigenetic Disparities in Cancer: Leveraging Pioneer Transcription Factors to Bridge Sequencing Gaps in racial ethnic Minorities
- Adetoun Musa, Texas Kidney Foundation, Unraveling the Complex Interplay of Neurobehavioral Disorders, Cardiometabolic Factors, and Genetics: An Integrative Analysis Using All of Us Datasets
- Andrew Nguyen, University of Maryland, Pericoronary Adipose Tissue Volume and Attenuation Can Predict Breast and Lung Cancer
- Yue Ning, Stevens Institute of Technology, Mitigating disparity of health outcomes via causal counterfactual predictions
- Howard Prioleau, Howard University, Enhancing Adverse Drug Event Detection in Minority Communities through Large Language Models, Text Generation, and Diverse Datasets
- Zahra Rahemi, Clemson University, Improving Risk Detection of Alzheimer's Disease and Related Dementia Among Diverse Populations Using Artificial Intelligence and Machine Learning (AI/ML) Models
- Korin Reid, Ellison Laboratories, Explainable Multimodal Graph Based Alzheimer's and Related Prediction Models for African American Patients using Large Scale Electronic Health Record Data
- Jaime Sesgundo, University of Nevada Reno, The Sleep Connection: Investigating the Impact of Sleep and Physical Activity on Alzheimer Disease Progression in Underserved Communities
- Kehlin Swain, Greens Health, Building AI models that predict diabetes complications by integrating clinical data and social determinants of health (SDOH)
- Xuan Wang, The University of Texas Rio Grande Valley, Building an AI-based visual Decision Support System for early disease detection with a built-in alert warning system for Minority Population
- Lucretia Williams, Howard University, Enhancing Cultural Relevancy in Behavioral Health Care for Racial-Ethnic Minorities through AI-Driven Data Analysis
- Elaine Yu, Vitalant Research Institute, Evaluating social vulnerability as a risk factor of type 2 diabetes subtypes and long COVID comorbidities: An application of machine learning in a retrospective patient cohort
- Anna Zamora-Kapoor, Washington State University, Leveraging artificial intelligence and machine learning to examine sleep disorders and chronic cardiometabolic conditions across racial and ethnic groups
- Ling Zhang, Northern Arizona University, A Mediation Model Approach to Uncover Risk Mechanisms and Treatment Barriers for Opioid Abuse Among Young Female Adults in Rural Areas
- Liang Zhao, Kennesaw State University, Unpacking Rural Disparities in Obesity through AI-enabled Social Determinants of Health Analysis