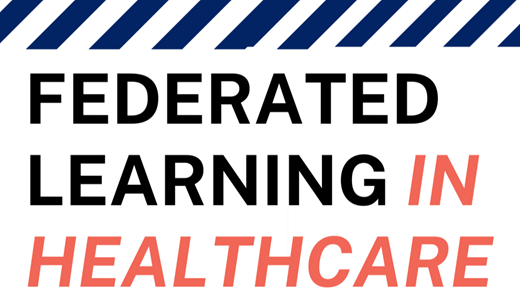
Federated Learning (FL) is transforming artificial intelligence by enabling decentralized model training across institutions without requiring direct data sharing.
This talk will begin with a brief review of foundational principles, structural components, and key methodologies, emphasizing its role as a privacy-preserving machine learning paradigm. Some key applications of FL in healthcare will be explored, including real-time patient monitoring, personalized treatment, broader medical research, and AI-driven drug discovery. Current implementations and studies demonstrate FL’s ability to enable multi-institutional collaboration while maintaining data privacy, allowing for improved model generalization, greater resource efficiency, and enhanced decision-making in clinical practice and research.
We will also review some of the challenges FL must overcome to maximize its benefits to health systems, such as data heterogeneity, model convergence issues, communication overhead, generalization limitations, and computational costs, which further complicate clinical adoption. As research advances and these challenges are addressed, FL has the potential to revolutionize collaborative AI in medicine, driving secure, data-driven healthcare innovation while safeguarding patient privacy.
Zoom link will be provided upon registration.
View the Flyer for more details
Wednesday, February 19, 2025 - 4:15 - 4:45 p.m. Eastern Time