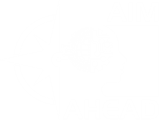
AIM-AHEAD Ethics and Equity
About
The AIM-AHEAD Ethics & Equity page is a central resource for discussing and exploring ethical issues within the AIM-AHEAD Program. Here, you can access expert insights, engage in meaningful conversations, and find valuable resources to guide your ethical decision-making.
-
Join Our Weekly Office Hours
Participate in live presentations on current ethical topics and bring your questions or concerns for group discussion and expert feedback. -
Engage in the Ethics Discussion Group
Connect with peers and ethics experts to share ideas and engage in ongoing conversations on ethics-related matters. -
Get Personalized Ethics Consultation
Receive tailored advice and support on ethical questions specific to your project or activity. -
Explore the Knowledgebase
Delve into a wide range of ethics-related topics to deepen your understanding and inform your work. -
Access AIM-AHEAD-Specific Ethics Resources
Discover materials designed specifically for the AIM-AHEAD community, addressing key ethical challenges in our field. -
Monthly Discussion Topics
Stay informed on emerging ethical issues with new topics introduced each month, encouraging continuous learning and dialogue.
See the Related Links for more information on all of these initiatives.
Ethics & Equity Events
Artificial intelligence in healthcare brings about a variety of issues related to ethics and equity which may be contextualized through an AI lifecycle. A lifecycle can provide a framework for thinking about these issues in a way that supports understanding how those issues arise and pathways to addressing them. This lifecycle-informed process synthesizes bioethics and healthcare ethics with technology ethics to facilitate interdisciplinary communication about AI and decision-making where potential benefits and possible harms can be weighed in an appropriate context as they are mapped across lifecycle steps from initial conceptualization to widespread use. The applicability of the lifecycle-informed process can be demonstrated by walking through cases documented in the literature related to real AI systems in a manner that can be adapted to other instances of AI systems.
Event Link:
https://us06web.zoom.us/j/85616593547?pwd=idDMYyXRnyqRy2FWn4bwcWABaNSk7b.1
Featured Speaker:
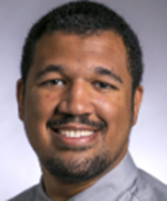
VUMC Department of Biomedical Informatics
Health care disparities in the United States continue to present significant challenges, with marginalized racial and ethnic groups often receiving disproportionately lower-quality care. A critical but underexplored aspect of these disparities is the engagement level of health professionals with patients’ electronic health records (EHRs) across different racial and ethnic backgrounds. This session presents a study of approximately 243,000 adult inpatients across two major U.S. academic centers, revealing that minoritized groups receive less EHR engagement compared to White patients. Using EHR access logs, we developed methods to quantify these disparities, highlighting the need for institutional awareness and targeted strategies to ensure equitable EHR engagement. Findings suggest that addressing these differences could be essential for improving patient care quality and outcomes across diverse populations.
Event Link: https://us06web.zoom.us/j/87381499158? pwd=0uLisz2hioXYb66xXDwki3RuDocgBY.1
Featured Speakers:
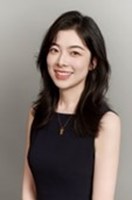
Vanderbilt University
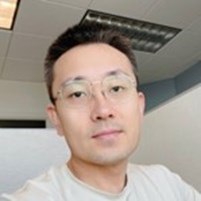
Vanderbilt University Medical Center
In federated clinical data networks, research queries and analysis code are distributed to each organization in the network. Those sites run the code locally and return only obfuscated aggregate counts and statistics to the investigators. The advantage of this architecture is that no patient-level data ever leave the organization. This enables organizations to retain control over their data, which lowers regulatory barriers to participation and addresses privacy concerns. We will describe examples of consortia using federated networks and discuss methods used to run artificial intelligence and machine learning algorithms across multiple sites.
Guest Speakers:
Griffin M Weber, MD, PhD
Associate Professor of Medicine and Biomedical Informatics
Beth Israel Deaconess Medical Center
Harvard Medical School
Tianxi Cai, ScD
Professor of Biomedical Informatics, Harvard Medical School
John Rock Professor of Population and Translational Data Sciences, Harvard T.H. Chan School of Public Health
Director, Translational Data Science Center for a Learning Health System (CELEHS)
Yong Chen, PhD
Professor of Biostatistics
Department of Biostatistics, Epidemiology, and Informatics
University of Pennsylvania
AI is not self-executing. We will discuss steps that need to be taken to ensure that it is implemented and used appropriately and that its effects are beneficial and minimize adverse sequelae.
Hosted by:
Ellen Clayton, MD, JD
Vanderbilt University Craig-Weaver Professor of Pediatrics, Professor of Law, Professor of Health Policy, AIM-AHEAD Co-Investigator, EEWG member
Laurie Lovett Novak, PhD, MHSA, FAMIA
Associate Professor, Biomedical Informatics, Vanderbilt University Medical Center, AIM-AHEAD Co-Investigator, EEWG member
Generative artificial intelligence (AI) has taken the world by storm and has teased promises of enhancing biomedical research and improving healthcare. The notion of generative AI is not new, but large language models (LLMs), chatbots, and multimodal translational technologies (e.g., text to video) have advanced rapidly over the past year. As with any new technology, there will be hope and hype, but poor design and management of such systems could induce and accentuate disparities, perpetuate biases, and cause safety problems for the management of patients. In this seminar, we will discuss what generative AI is (and is not), provide illustrations of this technology in biomedicine, illustrate potential pitfalls, along with opportunities for risk mitigation.
Hosted by:
Brad Malin, PhD
Accenture Professor, Vice Chair for Research Affairs, AIM-AHEAD Infrastructure Core
Dr. Nawar Shara
Founding Co-Director, AI CoLab, Chief, Research Data Science, MHRI, Co-Director of CBIDS, MHRI, Associate Professor of Medicine, GU, Director, BERD-GHUCCTS/CTSA, Co-Director of BI-GHUCCTS/CTSA
Without data, there is no modern artificial intelligence (AI). However, accessing healthcare data for purposes of building AI models is administratively difficult. There are many factors that limit healthcare data sharing on a broad scale, with privacy being a significant factor of general concern. Our society, and the organizations that function with it, have developed several strategies to balance healthcare data privacy with accessibility. These include, but are certainly not limited to, creating online data enclaves where users looking to build machine learning models must subject themselves to institutional oversight while performing analysis; enter into contractual agreements with data controllers, which can be onerous to establish, maintain, and manage; and subject data to de-identification practices meant to reduce the likelihood of individual reidentification. In this Ethics and Equity Seminar, we will walkthrough some of these strategies and provide examples current, real-world data sharing practices. We will also discuss how these strategies have impacted researchers engaged in AI development and the extent to which the existing array of datasets can (and cannot) support health equity research.
Rachele Hendricks-Sturrup, DHSc, MSc, MA, Functions within AIM-AHEAD's Infrastructure Core and EEWG co-chair
Brad Malin, PhD
Accenture Professor, Vice Chair for Research Affairs; Functions within AIM-AHEAD's Infrastructure Core
The use and role of race within health-related artificial intelligence and machine learning (AI/ML) models has become a subject of increased attention and controversy. The breadth of this topic spans both computational and socio-cultural aspects, going well-beyond the typical examination of bias and algorithmic fairness. Despite the complexity and multiplicity of issues that arise in use of race in AI/ML models for health, almost no holistic framing of this topic exists to guide stakeholders in interrogating and addressing the issues.
This discussion forum will:
- systematically explore the range of issues in use of race in AI/ML models at each step of the AI/ML lifecycle.
- examine several cross-cutting issues related to race in AI/ML models.
- Provide a set of ‘Points to Consider’ to guide inquiry and decision-making around this topic.
Participants are encouraged to bring and share questions and experiences around this topic. At the end of the discussion, participants should be equipped with a systematic way of comprehensively think about this topic, with usable guidance available to inform their own initiatives.
Martin C. Were, MD, MS, FIAHSI, FACMI, FAMIA
Professor of Biomedical Informatics, Professor of Medicine, Vanderbilt University Medical Center, Ethics & Equity Member
Chao Yan, PhD
Postdoctoral Research Fellow, Department of Biomedical Informatics Vanderbilt, Vanderbilt University Medical Center, Ethics & Equity Member
Trust is an essential component of healthcare. Patients who lack trust in healthcare tend to utilize healthcare less often and have worse health status than those who have more trust. The introduction to healthcare and increasing use of clinical artificial intelligence systems add further complexity to patient trust in healthcare within the clinical setting. Trust is also important for clinicians who will be using clinical AI systems, impacting the dissemination of those systems and how they are used. Much effort has been devoted to establishing patient and clinician trust in clinical AI , with attention to the goal of developing trustworthy clinical AI systems. This session will review the triadic relationship of patients, clinicians, and AI with a focus on trust, the factors that influence that trust, and the trustworthiness of clinical AI.
Benjamin Collins, MD, MS, MA, Vanderbilt University Medical Center, AIM-AHEAD Ethics Core
Malaika Simmons, MSHE Chief Operating Officer, National Alliance against Disparities in Patient Health (NADPH), AIM-AHEAD Ethics & Equity working group member
Artificial intelligence (AI) and machine learning (ML) technology design and development is often rapid and done without close examination and consideration of socio-humanitarian issues and complexities, including ensuring diversity in the AI/ML development and implementation workforce. This introduces risk for vulnerable and underserved populations subject to uses of the technology in consequential settings, such as health care and research. The AIM-AHEAD Ethics and Equity Workgroup (EEWG) recently developed Principles, a Glossary, and other tools to help facilitate engagement among stakeholders seeking to build equity in biomedical research, education, and healthcare through ensuring a diverse AI/ML development workforce. Join us for this virtual session to learn more about the collaborative process undertaken by the Workgroup to develop these tools, and engage in a robust discussion to contemplate next steps in implementing and refining the tools across low-resource AI/ML research settings.
Rachele Hendricks-Sturrup, DHSc, MSc, MA, Functions within AIM-AHEAD's Infrastructure Core and EEWG co-chair
Brad Malin, PhD
Accenture Professor, Vice Chair for Research Affairs; Functions within AIM-AHEAD's Infrastructure Core
Ellen Clayton, MD, JD, Vanderbilt University Craig-Weaver Professor of Pediatrics, Professor of Law, Professor of Health Policy, AIM-AHEAD Co-Investigator, EEWG member
Artificial intelligence and machine learning (AI/ML) involves the use of computer systems to model human cognitive functions like supervised and unsupervised learning and problem-solving. Today, AI/ML tools and devices are deeply embedded within our day-to-day health infrastructure, such as electronic medical records, diagnostic devices, and other tools used across health care and research settings. Also, technological advancements across a multitude of data sources have enabled the capture and continuous and precise analysis of sociodemographic factors, health behavior, disease, biomarkers, and other critical variables of health-relevance. Yet, there are many challenges and opportunities accompanying AI/ML implementation in health research and practice, which has driven a great deal of hype around the actual potential of AI/ML. In fact, the current lack of diversity of both data and researchers in the AI/ML field creates or poses risks to perpetuating harmful biases that may perpetuate health disparities and inequities across a growing range of health-relevant settings.
On April 6, 2023, the National Alliance Against Disparities in Patient Health partnered with Fisk University faculty and students to host a hybrid (in-person and online) roundtable discussion with faculty researchers, health care and policy practitioners, and community leaders to discuss the realities and hype surrounding AI/ML use in health research.
Joining Rachele Hendericks-Sturrup were expert panelists from Vanderbilt University, Meharry Medical College, and the Southern Nevada Black Nurses Association.
![]() |
Rachele Hendricks-Sturrup, DHSc, MSc, MA, who functions within AIM-AHEAD’s Infrastructure Core and co-chairing AIM-AHEAD’s Ethics and Equity Workgroup |
![]() |
Benjamin Collins, MD, MA; Vanderbilt University Medical Center Physician and Postdoctoral Fellow in Ethics, Legal, and Social Issues of Artificial Intelligence in Healthcare; AIM-AHEAD Co-Investigator; AIM-AHEAD Ethics and Equity Workgroup member |
![]() |
Ellen Clayton, MD, JD; Vanderbilt University Craig-Weaver Professor of Pediatrics; Professor of Law; Professor of Health Policy; AIM-AHEAD Co-Investigator; AIM-AHEAD Ethics and Equity Workgroup member |
![]() |
Lauren Edgar, DNP, RN, MSN-Ed.,FNP; Former President, Southern Nevada Black Nurses Association; AIM-AHEAD Leadership Fellow |
![]() |
Millard Collins, M.D., FAAFP; Dr. Frank S. Royal Endowed Chair; Professor, Department of Family & Community Medicine; Meharry Medical College |
The roundtable discussion was a tremendous success, resulting in over 200 Fisk University students in attendance receiving professional development credits toward graduation. Students and faculty in attendance expressed excitement and enthusiasm as well as eagerness to learn about career options and pathways in AI/ML health research, how AI/ML intersects with health equity, how AI/ML is bring used to advance the practice of medicine and health research, ethical issues accompanying AI/ML research implementation in underserved health care settings, and more. View the full recording of the roundtable discussion below.
Fisk AI/ML Symposium April 5, 2023
Following the event, on April 5, 2023, Dr. Hendricks-Sturrup presented the AIM-AHEAD Ethics and Equity Principles to several esteemed Fisk University students during their 2023 Honors Convocation ceremony. View the full recording of the 2023 Honors Convocation below.
https://www.youtube.com/live/-QGMgVFF8f4?feature=share
Very special thanks to Sheanel Gardner (Fisk University, ‘23), Dr. Sajid Hussain, and Dr. Phyllis Freeman at Fisk University for providing coordination, operational, and outreach support for this event to facilitate its community impact and success.
To learn more about the AIM-AHEAD Ethics and Equity Workgroup, contact Dr. Rachele Hendricks-Sturrup at hendricks-sturrup@nadph.org or Dr. Bradley Malin at Bradley.malin@vanderbilt.edu.
To learn more about AI/ML research opportunities at Fisk University, contact Dr. Sajid Hussain at shussain@fisk.edu.
To learn more about activities and research opportunities within the AIM-AHEAD Infrastructure Core, contact Dr. Bradley Malin at Bradley.malin@vanderbilt.edu or Dr. Alex Carlisle at carlisle@nadph.org.
Without data, there is no modern artificial intelligence. However, getting access to data, and particularly data about a person’s biology or healthcare, can be difficult. Many factors limit health data sharing on a broad scale, with privacy being one of the principles most voiced as a concern. Our society, and the organizations that function with it, have devised a number of strategies for managing the tradeoff between health data privacy and accessibility. These include, but are certainly not limited to,
- creating online data enclaves where users are limited by the program languages and computing infrastructure support (e.g., pay per compute in the cloud),
- entering into contractual agreements with data controllers, which can be onerous to establish, maintain, and manage, and
- subjecting data to de-identification practices that reduce the likelihood that the identity of the individuals to whom the data corresponds will be recognized.
In this ethics discussion forum, we will walk through some of these strategies, as well as examples of how data sharing practices have been realized historically. Along the way, we will discuss how the tradeoffs inherent in these strategies can affect,
- which researchers have access to these data and
- the extent to which the resulting datasets can (and cannot) support health equity research.
Discussion host:
Bradley Malin, Ph.D., Vanderbilt University Medical Center
The potential for artificial intelligence and machine learning (AI/ML) to address health equity in an ethical manner is an ongoing discussion, with both debate and uses cases across a growing range of health-related scenarios. Centered within this debate are broad considerations for convened institutions and individual stakeholders who are seeking to fulfill this potential collaboratively, meaningfully, and effectively, such as AIM-AHEAD. Members of the AIM-AHEAD Ethics SubCore will present, on behalf of the AIM-AHEAD Ethics and Equity Workgroup, the AIM-AHEAD Ethics and Equity Principles and Glossary to drive a moderated discussion around challenges and opportunities to implementing the principles and glossary among diverse institutions and collaborators within the Consortium. Individuals who attend will also have the opportunity to sign up to participate in a 1:1 interview with members of the AIM-AHEAD Ethics and Equity Workgroup to discuss their personal views and perspectives about the Principles and Glossary and provide feedback on opportunities to strengthen the Principles and Glossary.
Discussion hosts:
![]() Rachele Hendricks-Sturrup, DHSc, MSc, MA National Alliance Against Disparities in Patient Health (NADPH) AIM-AHEAD Ethics and Equity Workgroup Co-Chair |
![]() Ellen Wright Clayton, MD, JD Craig-Weaver Professor of Pediatrics, Professor of Law Center for Biomedical Ethics and Society Vanderbilt University Medical Center AIM-AHEAD Ethics and Equity Workgroup Member |
![]() Malaika Simmons, MSHE National Alliance Against Disparities in Patient Health (NADPH) AIM-AHEAD Ethics and Equity Workgroup Member |
Moderated by Benjamin Collins, MD, MA and Rachele Hendricks-Sturrup, DHSc, MSc, MA
Peer-reviewed literature and the broader media continue to highlight the risks and challenges as well as the opportunities and benefits of artificial intelligence and machine learning (AI/ML) development, translation, and implementation in health research and practice. For example, recent literature [1] has discussed serious health equity concerns regarding the use of race correction in clinical algorithms spanning across multiple medical specialties including cardiology, cardiac surgery, nephrology, obstetrics, urology, oncology, endocrinology, and pulmonology. Race correction can result in problems such as improper screening/monitoring cadence; inaccurate estimates of organ function, risk adjustments and profiling, and disease risk. Recent news stories have also covered issues around the use of algorithms and machine learning models that inaccurately and inappropriately predict stroke risk in Black (versus White) patients [2]. Lastly, as OpenAI released its ChatGPT chatbot, institutions are considering the use and application of ChatGPT in clinical practice and education with great controversy [3]. The AIM-AHEAD Ethics Sub-Core will host an AIM-AHEAD Ethics Discussion Series about these issues and others, with a goal to drive moderated discussion, ideas, and collaboration within the AIM-AHEAD community on the ethical development, use, and implementation of AI/ML in health research and practice.
[1] Vyas DA, et al. Hidden in plain sight – reconsidering the use of race correction in clinical algorithms. New England Journal of Medicine. 2020; 383: 874-882. https://www.nejm.org/doi/full/10.1056/NEJMms2004740
[2] Castillo A. Tools to predict stroke risk work less well for Black patients, study finds. Stat News. February 22, 2023. https://www.statnews.com/2023/02/22/stroke-risk-machine-learning-models/
[3] Doshi RH, Bajaj SS. Promises - and pitfalls - of ChatGPT-assisted medicine. Stat News. February 1, 2023. https://www.statnews.com/2023/02/01/promises-pitfalls-chatgpt-assisted-medicine/